Simplify Trending Using Percentile Calculations
In oil analysis, we often have to interpret a change in one parameter relative to a change in another to reach a meaningful conclusion. For example, a rise in wear levels combined with a corresponding decrease in zinc might tell us that wear is increasing due to the loss of anti-wear additive protection. Further "reading between the lines" using viscosity, TAN and infra-red analysis data might tell us whether the reduced anti-wear protection is the result of the addition of the wrong oil or additive depletion, and so forth.
Unfortunately, while these parameters are all important to our analysis, they use widely varying units and each has its own degree of random variation (data fragility). That makes charting the values over time on a common graph difficult. Also, different alarming logic is applied to the different parameters. One way around this problem is to trend statistically derived "percentile" ratings instead of actual parameter values. This simple technique yields the following benefits to the oil analyst:
• All parameters can be reviewed on a common trend graph using common units (the percentile) making it easy to see what is rising and what is falling simultaneously, facilitating the process of reading between the lines (see Figure 1). In the figure, it is apparent that viscosity is falling while particle count and iron have risen dramatically, suggesting that the wrong oil might have been added to the machine and that has induced high wear rates.
• Common alarms can be set for all parameters and displayed on a common graph. For example, one standard deviation might represent a caution, while two might suggest a critical situation (see Table 2).
• The noise effects of normal variation are factored out because each parameter's percentile calculations are based upon its own standard deviation.
• Percentiles can be understood by anyone (including management). Particle count, mg KOH/g of oil, etc. are not so obvious to the untrained observer.
• Non-oil analysis parameters ranging from vibration overalls to skirt length can easily be incorporated into the graphics and, thus, into the analysis and decision processes.
• The technique is fast and easy.
Here is how to translate oil analysis data into percentiles:
• Determine, using historical data, the average value (mean) for each parameter (see Equation 1).
• Calculate the standard deviation for each parameter using the same data set used to calculate the mean (see Equation 2).
• Generate a "Z-Score" by subtracting the mean value from the current reading and dividing by the standard deviation (see Equation 3). This number tells you how many standard deviations you are over or under the mean value.
• Using cumulative normal distribution tables (most commercially available spreadsheet programs generate a cumulative distribution value for a given Z-Score).
• Present the normal distribution value as a percentile value.
Click here for a list of equations.
For example, suppose a machine has a mean iron level of 15 ppm and a standard deviation of 3 ppm. An observed value of 18 ppm would yield a Z-Score of 1, or one standard deviation greater than the mean. The 18 ppm value would occur at the 84th percentile. Had our observation occurred at the mean (15 ppm) our value would be the 50th percentile. The table illustrates where various Z-Scores occur on the cumulative normal distribution curve.
This and other techniques can be effectively applied to simplify oil analysis data and ease the diagnostic process. Try variations on the percentile theme like using a 10 sample moving average and standard deviation in place of the fixed values where it is appropriate. Such simplification is important for oil analysis to gain acceptance into the mainstream of the decision making process.
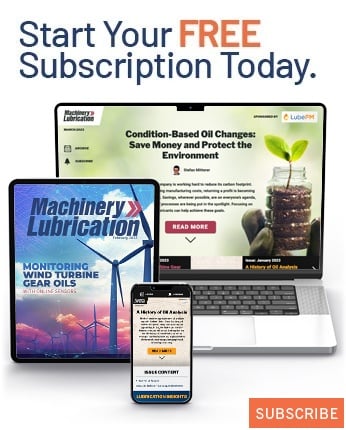