Reliability engineering as a discipline really found its feet during the 1960s as commercial aviation started to take off. Indeed, many of the fundamental tenets of modern-day reliability engineering – tools such as Reliability-Centered Maintenance (RCM), failure modes effects and criticality analysis (FMECA), and root cause analysis (RCA) – were developed to help improve safety in commercial aviation. In the world of aircraft maintenance and operations, reliability is all about risk management: applying systematic tools to identify weaknesses in systems or processes to help eliminate or minimize the likelihood of occurrence.
But while these tools have been highly effective in insuring that, today, commercial flight is by far the safest mode of transportation, there is always a risk (albeit small) that a catastrophic event might happen. As a frequent flyer, I’m comfortable that every effort has been taken to keep me safe but understand that there’s a finite opportunity that a catastrophe could occur.
PdM does not change the probability of failure, but rather the consequence.
In industry, reliability engineering is also about risk management. And while the stakes are usually lower than in the aviation world, the same underlying principles of cause and effect apply, making management of risk a top priority. Just like commercial aviation, systematic engineering can be applied to help minimize risk. For example, just like the critical flight control systems on an aircraft, we might elect to deploy redundant pumps in a critical process bottleneck area. But while redundancy will certainly help lower the risk, there is always a finite chance that both main and backup systems could fail.
Similarly, we can systematically calculate the required grease intervals and volumes for each point in the plant; and while there’s little doubt that this will reduce the likelihood of a lubrication-related failure, there’s no guarantee that we will completely eliminate the risk.
Risk can be defined very simply as:
Risk = Probability of Failure x Consequence of Failure
Take, for example, a critical flight control system on a commercial aircraft. While through the application of good design and engineering the probability of a flight control system failing is low, the consequence is so severe that redundant systems are deployed, such that a single event cannot compromise the aircraft. By contrast, the gear motor that powers the jet bridge drive system used to ferry passengers onto the aircraft likely has a much higher probability of failure, but the consequence of failure is so low that we would probably consider this a run-to-fail component.
In industry, maintenance and reliability engineers deploy two basic strategies to insure asset reliability: predictive maintenance and proactive maintenance. Loosely defined, predictive maintenance involves the application of predictive tools such as inspections, ultrasonics, vibration analysis and wear debris analysis to try to identify early warning signs of impending failure. Proactive maintenance, on the other hand, involves the use of feedback tools to insure possible causes of failure – such as excess particle contamination, lubricant degradation, machine imbalance or misalignment – are within “safe” tolerances.
So, how does predictive and proactive maintenance apply to risk management? Using our definition of risk in this article, it should be obvious that predictive maintenance doesn’t address the probability of failure, but rather the consequence, while proactive maintenance targets the probability of failure, not the consequence.
To understand this, consider using vibration analysis to monitor bearing defect frequencies, a purely predictive activity. No matter how often we take a vibration reading (even monitoring continuously), we can’t influence the probability of failure (how long the bearing will last); we can only hope to find the problem early enough that corrective action can be planned for a time that is least disruptive and costly to the organization. With predictive maintenance, we can control the consequence of failure (cost per event) but not the probability.
Conversely, proactive maintenance targets the probability of failure but not the consequence. As an example, consider proactively improving the levels of fluid cleanliness in a poorly maintained servo-controlled hydraulic system. While it is likely that improved cleanliness will improve the probability of failure (mean time between failure), were the machine to fail even with improved cleanliness, the consequence of failure (cost to the organization) would be unchanged.
To be successful, we need to control risk from both fronts: probability of failure and consequence of failure. We shouldn’t be content with just finding failures (predictive maintenance). Rather, we should seek to eliminate causative factors through the application of proactive maintenance.
As always, this is my opinion; I’m interested to hear yours.
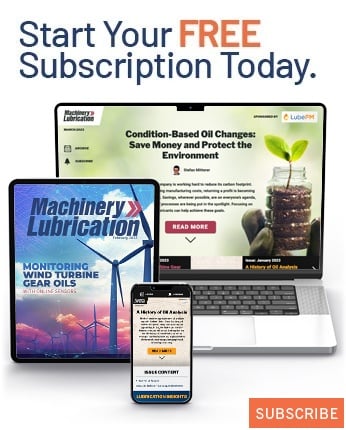