
In my last column, I discussed the need to optimize preventive maintenance activities in order to:
-
eliminate tasks that fail to add value or actually create problems;
-
reduce the ambiguity of maintenance tasks; and,
-
avoid "PM stacking", where condition monitoring tasks are incorporated in the plan, but we fail to eliminate the time-directed tasks they're intended to replace.
In this column, I will detail the logic and the process for creating PM plans. This article includes two parts. First, we'll discuss the decision-making approaches you might apply and the conditions under which a particular approach is chosen over another.
In the second part, we'll explore the process of building an enterprise-level PM plan for various equipment classes and then determine how to apply those plans at the site- and equipment-specific levels.
Three Approaches
In general, reliability engineering practice offers us the following three approaches to building up a PM plan:
-
Reliability-Centered Maintenance (RCM) approach
-
Failure reporting and corrective action system (FRACAS) approach
-
Judgment-based approach
Let's begin with the RCM approach.
RCM is a systematic process for developing an optimized maintenance policy for a system that is being designed and built. In essence, if we do not have any history on a system, we systematically assess risk associated with specific failure modes to determine possible consequences, evaluate existing controls and assign a risk priority number (RPN).
This process is completed on a failure modes and effects analysis (FMEA) worksheet, and specific controls are defined (e.g. maintenance tasks, redesign, redundancy/critical spares, etc.) to mitigate risk, thus reducing the risk and the associated RPN. RCM works. The only real issue is that the process is slow and expensive.
Figure 1. Decision matrix helps select the best PM optimization process.
In reality, for most of the equipment in most manufacturing plants, you don't have to gather around a table and speculate what might go wrong; the failures are occurring and have been occurring for some time.
In my opinion as a reliability engineer, a FRACAS-based approach is much more efficient. By systematically collecting data about failures and their causes and associated effects, we can more rapidly and accurately assess risk and formulate an optimized maintenance strategy based on actual problems you're seeing in the plant.
FRACAS-based PM optimization is my preferred method. Data enables us to truly assess the consequences of failures on a mode-by-mode basis, apply standard reliability engineering methods to quantify failure frequency (e.g. mean time between failures [MTBF] and mean time to failure [MTTF]), and evaluate the risk profile as a function of time using tools like Weibull analysis.
The third approach is judgment-based. In the absence of good data, it is sometimes preferred to simply rely on the judgment of experienced maintenance and systems engineers to formulate a plan based upon their collective experiences and recollections. While not as accurate as the FRACAS-based approach, it's often the best choice if the equipment in question is neither operationally critical, expensive to replace or repair, nor a known bad actor.
Deciding on an Approach
So, how do we decide on the approach? Two factors drive the decision: equipment/process criticality and the quality of FRACAS data.
Referring to Figure 1, if the system criticality is high and the quality FRACAS data is high, we employ the FRACAS method for routine maintenance tasks and supplement with RCM to analyze high-impact, low-frequency-of-occurrence failure modes - what Deming referred to as "rare events."
FRACAS is the primary driver here as it is the most reliable decision support method available. However, where the criticality is high but the availability of high-quality FRACAS data is low, we must depend upon the tried-and-true - albeit somewhat arduous - RCM methodology of employing inductive-reasoning methods to evaluate what might go wrong.
Conversely, where system criticality is low, we needn't really ever apply the RCM. If the quality of our FRACAS data is high, we simply let historical observations drive the PM optimization process. Where criticality is low and the quality of our FRACAS data is poor, we apply judgment-based methods for optimizing the PM plan.
In all instances, we employ "dollarized" FMEAs to log the findings of our analysis and associated decisions. The FMEA represents the risk management log for a manufacturing system. Machines come and go, but the FMEA should provide an organized historical summary of all risk management decisions across the life cycle of the targeted manufacturing process.
Also, in instances when the quality of FRACAS data is poor, develop systematic data collection systems to drive PM plan refinement and continuous improvement. Your FRACAS process should be based upon a standardized methodology that incorporates standardized taxonomies of failure modes and causes.
Building the Master Plans
Once you've settled on an appropriate decision process, it's time to start building the PM plans for a particular machine class or sub-class. We call these master plans. A master plan should actually be built up as a compilation of component-level master plans that are assembled into a PM master plan for the specified class/sub-class of equipment.
For example, a motor-driven pump of a particular type requires a specific PM master plan. But the general PM for the motor should be the same for all motor-driven systems, so the PM plan for the motor driving the pump can be repurposed to other motor-driven equipment assets. Here are a couple of things to consider when creating component-level master plans:
1. Set up component-level master plans based on equipment demographics. Using the electric motor example, there are specific tasks that are applicable based upon equipment design and operating context variables, including the following:
a. System criticality
b. Horsepower
c. Motor type (e.g. AC, DC, VFD)
d. Accessibility for maintenance
e. Bearing type
f. Shaft orientation (horizontal, vertical, offset)
g. Lubrication type/system.
2. Incorporate attribute variables into the PM plan. Attribute variables are the meat of a task or procedure. Defining them eliminates task ambiguity. For instance, we frequently see tasks that read "check system pressure" or "verify belt tension." Where possible, define the attribute variables with an acceptable range statement, a "not to exceed" statement and/or a "not to fall below" statement. In many instances, a PM task requires the specification of several attribute variables.
3. Create visual PMs and a visual plant. A picture is worth a thousand words. Adding pictures to tasks helps the technician find PM activity points on the machine and, where attribute variables can't be defined, a picture can demonstrate acceptable vs. unacceptable conditions (particularly useful for visual inspections). Wherever possible, place required information at the point at which the work is done - either at the machine or loaded into handheld data collectors or PDAs.
4. Develop system-level PM tasks into the class-level or sub-class-level master plan. While the buildup of the plan from lower-level component plans will standardize much of the work that needs to be done, there are overarching system-level tasks that must be included into each class/sub-class master plan.
5. Create standard parts and labor time estimates for each task. The sum of the projected PM costs should be compared to the current actual spend to determine the cost benefit analysis. If the cost is about the same or more than the current spend for the asset class/sub-class, then justify the changes based upon improved reliability. In most cases, the projected cost will be less than the current spend for preventive maintenance, which produces a cost reduction. (We frequently see a 25 percent or more reduction in direct PM costs as well as improved reliability.)
6. Create standard task assignments for each task. Typical assignment categories include:
a. Mechanical maintenance
b. E&I maintenance
c. Operator/TPM care
d. Predictive maintenance (PdM) technician
e. Contractor
f. Etc.

Figure 2. Your PMO process must strike a balance between standardization and customization and provide an auditable trail between equipment class/sub-class master plans, site plans and equipment plans.
Assigning the Master Plan
Once the master plan is created, it is time to assign it. For multi-plant operations, there may be site-specific changes (see Figure 2). For instance, there may be specific safety or environmental requirements at one plant that don't apply to all.
This is particularly true if the organization operates plants in multiple countries. Likewise, task assignments may need to vary from site to site. Some plants may utilize multi-skilled craft or have bargaining unit restrictions on the assignment of specific tasks to operators.
Also, some plants tend to rely more or less on contractors for PdM or other work. Likewise, when assigning PM master plans to specific machines, you'll need to define the attribute variables specific to that application.
In some instances, tasks may need to be added, deleted or modified to address variations in equipment design, operating context and/or environmental conditions. You'll need to create your master plans within a database application that affords you the flexibility to make these changes and track them so you have an auditable trail between the master plan, the site plans and the equipment plans.
Tough, But Worth It
PM optimization is serious business. It provides you with the opportunity to both cut cost and improve reliability by eliminating unnecessary tasks, removing task ambiguity and standardizing on practices enterprise-wide - repurposing and leveraging your firm's know-how and intellectual property. But, PM optimization must be done systematically, striking a balance between standardization and customization.
Plan to succeed. Do your homework and seek help where required.
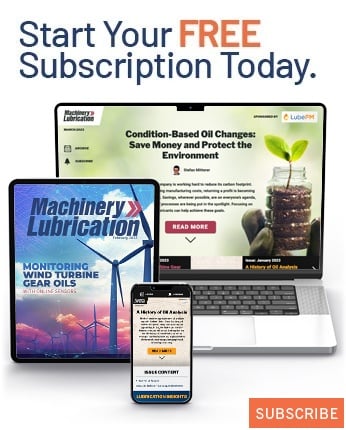